How does generative AI work?
Explore the inner workings of Generative AI, from data collection and training to real-world applications.
How does generative AI work?
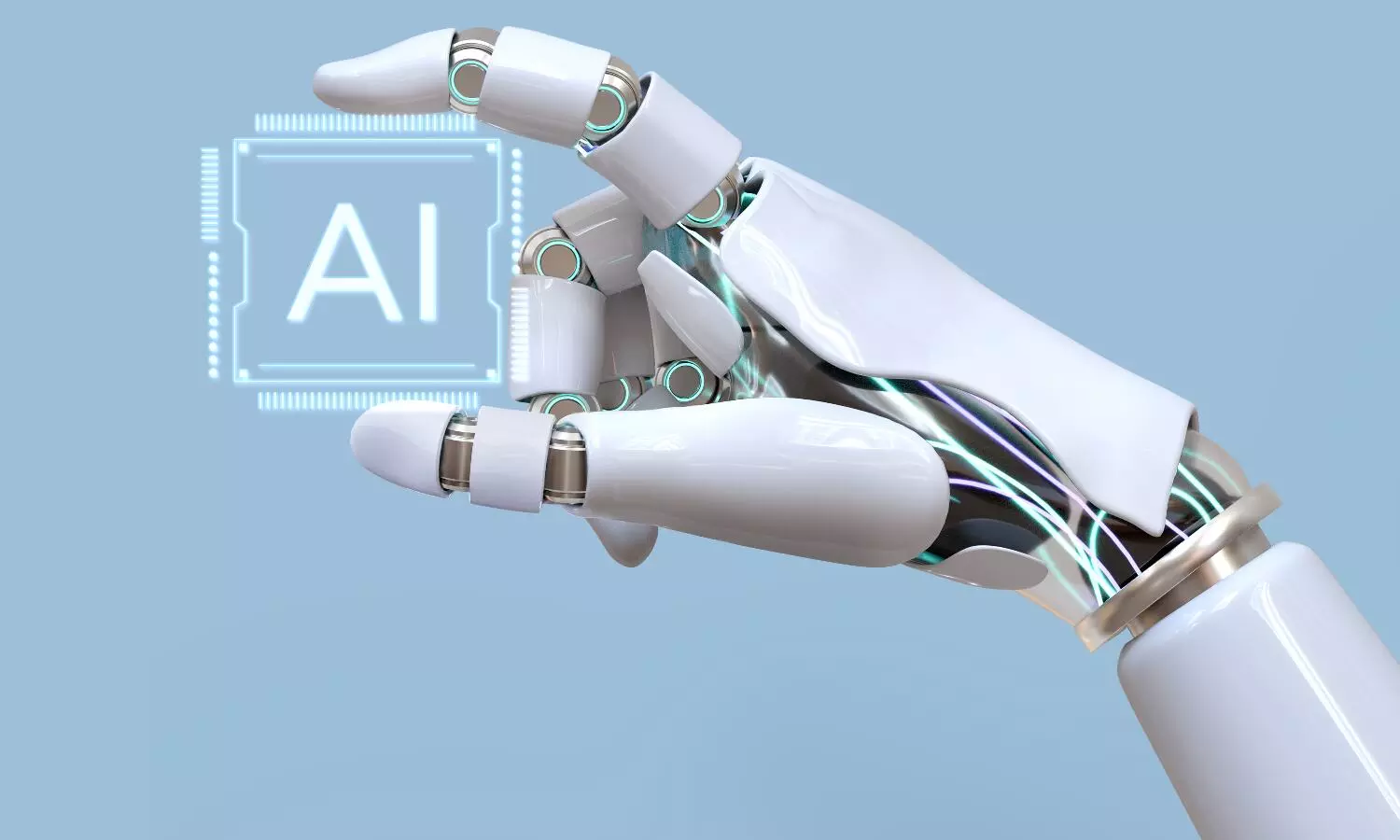
Generative AI is quickly becoming a transformative technology, allowing machines to create new, original content from scratch. This can range from images and text to music and videos, all created by models that learn from vast amounts of existing data. But what makes this possible? How does generative AI actually work? Let’s dive into the processes, models, and applications that make this fascinating technology tick.
What Is Generative AI?
Generative AI is a branch of artificial intelligence designed to generate new content based on patterns and structures found in existing data. Unlike traditional AI, which often focuses on recognising or classifying information, generative AI learns from data to produce original outputs, such as text, images, or music. These AI models are typically built on deep learning and neural network techniques, enabling them to analyse large datasets, recognise patterns, and create outputs that resemble human-generated content.
For example, a generative AI model trained on thousands of images of landscapes could learn the characteristics of those images and then generate entirely new, yet plausible, landscape images on its own. Similarly, generative AI can also be used to create meaningful text, craft new music, or even generate video game levels.
The Process Behind Generative AI
Generative AI operates through several key steps, starting from collecting data to generating new content. Below are the crucial stages involved in its operation:
1. Data Collection
The journey begins with gathering a large, diverse dataset. This dataset should closely match the type of content the AI model will create. For instance, if the goal is to generate realistic images of cats, a collection of thousands of cat images would be gathered. This data is then fed into the model to teach it about patterns and relationships.
2. Model Training
Once the data is collected, the next step is training the model. Generative AI typically employs deep learning techniques, where the model analyses the dataset to learn the underlying features. One popular approach is using Generative Adversarial Networks (GANs) or Variational Autoencoders (VAEs). These models help the AI understand complex data distributions, learning how different elements in the data relate to one another.
3. Latent Space Representation
After training, the model creates a mathematical representation called latent space. This abstract representation captures the essence of the training data. It allows the AI to understand the key features of the content it has learned, compressing the information in a way that makes it possible to generate new examples later.
4. Content Generation
At this stage, the AI can generate new content by sampling from the latent space. For instance, when generating an image, the model will pick a point in latent space and decode it back into an image that resembles the ones it learned from during training. This is where the AI gets creative-drawing from its understanding to produce something new.
5. Iterative Improvement
Generative models typically undergo an iterative refinement process. Initially, the AI might produce imperfect results, but through continuous training and feedback, it can improve over time. This iterative process helps the AI generate outputs that are more realistic and closer to the quality of human-generated content.
Key Components of Generative AI Models
Generative AI relies on several crucial components to work efficiently and effectively:
- Generative Models: These algorithms, like GANs and VAEs, are designed to learn data patterns and generate new content. GANs use a generator and a discriminator network, where the generator creates synthetic content, and the discriminator evaluates it. Over time, the generator improves its output based on feedback from the discriminator.
- Neural Networks: Deep learning models like Convolutional Neural Networks (CNNs) and Recurrent Neural Networks (RNNs) are used to process data. These networks help the AI understand complex patterns in images, text, or even sequential data like music.
- Latent Space: This lower-dimensional representation is crucial for generating new data. By manipulating the latent space, generative models can create variations of the original content learned from the training data.
- Fine-Tuning: Once a model is trained on general data, it can be fine-tuned for more specific applications, such as creating medical images or generating legal text. This fine-tuning allows the AI to specialise in particular tasks.
Types of Generative Models
Several types of generative models are employed depending on the nature of the task at hand. Here are some common ones:
- Generative Adversarial Networks (GANs): These models consist of two networks-the generator and the discriminator-that compete with each other. The generator tries to create convincing content, while the discriminator tries to identify whether the content is real or fake. Over time, this competition helps improve the quality of the generated content.
- Variational Autoencoders (VAEs): VAEs work by compressing data into a latent space and then reconstructing it. They learn to generate new content by sampling points in this latent space, making them useful for tasks like image generation and data augmentation.
- Autoregressive Models: These models, like Recurrent Neural Networks (RNNs), predict the next data point in a sequence based on previous ones. They are commonly used for generating text, music, or other sequential data.
Use Cases of Generative AI
Generative AI is revolutionising various fields, with a multitude of practical applications:
1. Content Creation
One of the most significant uses of generative AI is content generation. For instance, AI can write articles, generate social media posts, or create blogs by learning from vast amounts of text data. This is increasingly being used in industries like marketing, journalism, and entertainment.
2. Image Generation
With tools like GANs and VAEs, AI can create highly realistic images. This technology is employed in industries ranging from fashion design to video game development, where it’s used to generate assets like characters, environments, and objects.
3. Music and Art Creation
Generative AI is also making waves in creative industries. AI-driven models can compose original music or generate art that mimics particular styles or genres. These models help artists and musicians brainstorm new ideas or produce unique content quickly.
4. Data Augmentation
In machine learning, generative models can create synthetic data to supplement existing datasets. This is especially valuable when there’s a shortage of labeled data for training models, as it allows for better performance and generalisation.
5. Medical Imaging and Drug Discovery
In healthcare, generative AI is used to generate synthetic medical images for training diagnostic models or even create new molecular structures for drug discovery. This has the potential to significantly speed up research and development in the medical field.
Challenges and Ethical Considerations
Despite its incredible potential, generative AI also raises important ethical concerns:
- Deepfakes: One of the more controversial applications is the creation of deepfake videos, which manipulate real footage to create fake representations. This raises issues around misinformation and privacy violations.
- Bias: Since generative models learn from data, any biases in the training data can be replicated in the generated content. This is a critical challenge, particularly in areas like hiring algorithms and criminal justice.
- Quality Control: Although generative AI models are capable of producing impressive outputs, there is still a risk of generating low-quality or unrealistic content, which requires constant refinement.
Conclusion
Generative AI is an exciting and rapidly evolving field that holds immense promise for creative industries, data analysis, and beyond. By understanding how it works and the models that drive it, we can better harness its capabilities and address its challenges. Whether you're a content creator, a researcher, or just an AI enthusiast, it’s clear that generative AI is poised to reshape how we interact with technology in the years to come.